Me-too drugs and the butterfly effect
LONDON, Sept. 17, 2015 /PRNewswire/ — With regulators, Health Technology Assessment agencies and payers demanding demonstrable evidence of value it is becoming increasingly difficult for Pharma to not only get new products approved, but also to negotiate premium reimbursement or ensure continuing reimbursement for licensed brands. Consequently, success of a new launch or an established brand is invariably reliant upon demonstrating value-differentiation. Accordingly, a brand must exhibit previously-unidentified comparative superiority within an approved indication whereby it uniquely fulfils an unmet clinical need, or provide a therapeutic benefit that cannot be matched by competing brands.

Whereas big data is perceived as the panacea for identifying new value, in practice, locating real world evidence within big data is often like searching for a white soft ball in snow.
"Real world outcomes are more deterministic than random," comments Dr Keith McCormack, founder and Research Director of McCormack Pharma, adding that… "So we need to understand what kind of world made these data."
And in 1983, he built the technology platform that became known as CEME which, by discovering previously-unknown relationships within natural language text databases was consistently accurate in identifying patients who intrinsically show a maximal response to a particular drug. That is, outcomes from text databases showed where to search for real world evidence within observational big data.
Not by chance, CEME has its origins within chaos theory. For a deterministic system that is chaotic, outcomes are not predictable and are sensitive to starting values. Likewise, in the quasi-chaotic system of structure-activity relationships, by comparison with behaviour in a chaotic system differences in starting values also result in significant differences in outcomes; but because differences in molecular structure are constant, then the effects of classical iterative amplification of small errors upon final states are similarly fixed. CEME displays exquisite sensitivity in differentiating drugs that share the same approved indication and accordingly it has the potential to identify new value for a given drug.
For example, in locating a patient subpopulation that intrinsically show a maximal response to a specific drug, a basic axiom of the CEME process is that as a subpopulation, the existence of these patients is coded by some previously-unknown relationship (association) between the drug and data that characterizes a selected therapeutic (approved) indication. Collectively, text databases that include Medline, Embase and Biosis represent an invaluable repository of previously-unknown relationships which by definition are not obvious during normal use.
However, as a tool for discovering previously-unknown relationships, association rule learning is a well-studied data mining task that was popularised by the celebrated 1993 publication of Rakesh Agrawal and coworkers.
And it must be emphasized that the use of keywords to search text databases is "information extraction" and not mining. Moreover, by comparison with the kind of data stored in structured databases, natural language text is unstructured, amorphous, and difficult to mine using traditional algorithms.
Regardless of the origins of association rule learning, and notwithstanding the considerable difficulties in mining natural language text, in the 1980s McCormack Pharma pioneered the use of antecedent surrogate variables in association rules learning before such methods were widely used or even understood; moreover, at that time the field of text mining was barely nascent. Critically-dependent upon the chosen field, it is the selection of antecedent surrogate variables that directs the probe of natural language text for the discovery of new and interesting associations that otherwise would remain undiscovered.
More about McCormack Pharma and CEME
Case histories can be viewed at www.mccormackpharma.com
Company contact: Keith McCormack PhD
Research Director
+44 (0) 20 8359 1218
Relevant link: www.mccormackpharma.com
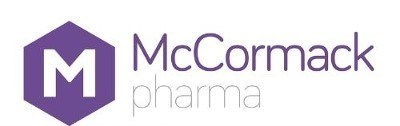
Photo – http://photos.prnewswire.com/prnh/20150916/267172
Logo – http://photos.prnewswire.com/prnh/20150916/267173LOGO